#wannabeeeeeee the best DataScientist
SVM + Python_Code 본문
728x90
◎ Support Vector Machine(SVM)
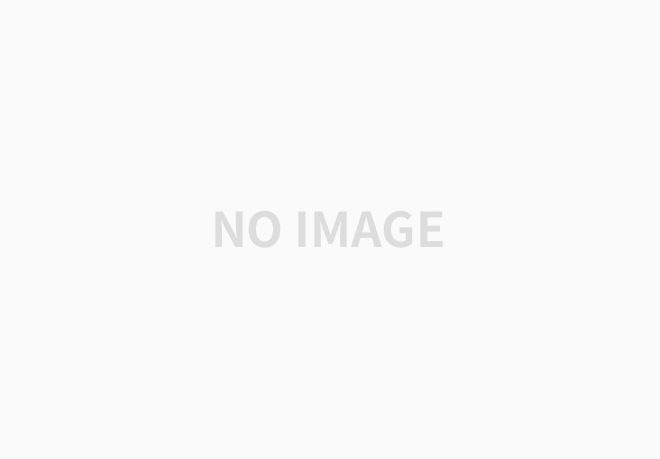
◆ 종속 변수 데이터 형태에 따라 둘로 나뉜다.
◇ 범주형 변수 : Support vector classifier
◇ 연속형 변수 : Support vector regression (SVR)
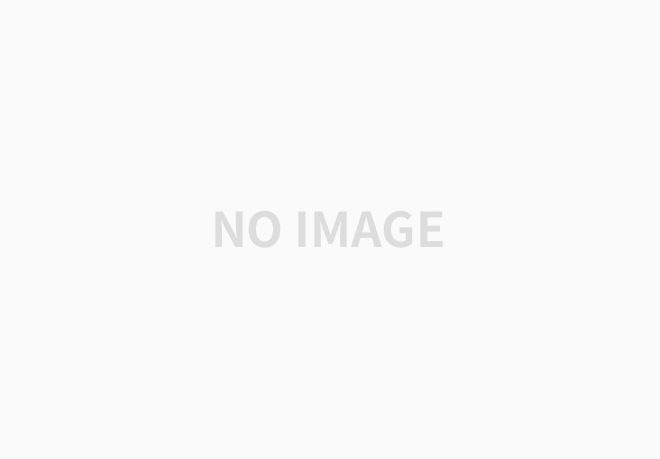
◆ SVM 계산
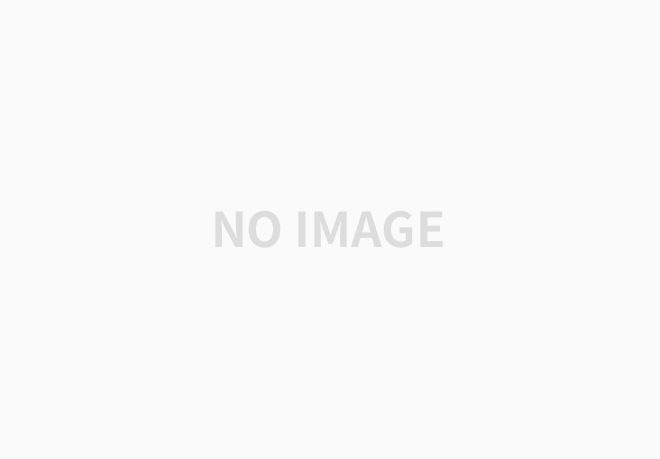
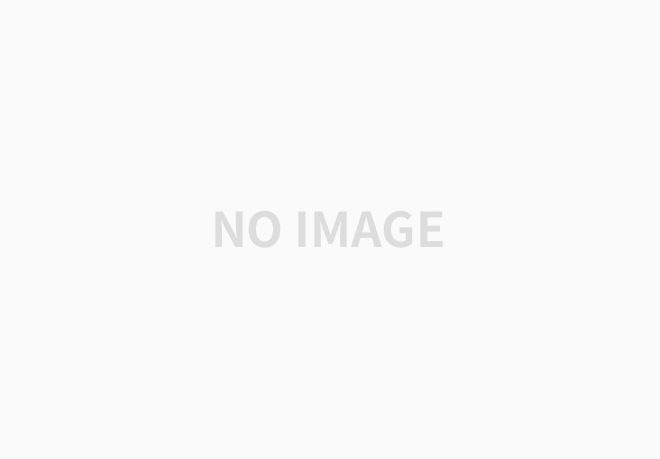
◆ SVM with Kernel
- 선형 관계가 아닌 경우에 사용
- 비선형 구조의 데이터를 fitting할 때, Kernel을 사용할 필요가 있음
- 차원이 높아짐에 따라 추정해야 하는 모수의 개수가 많아짐에 따라 Test error가 높아지는 현상 발생
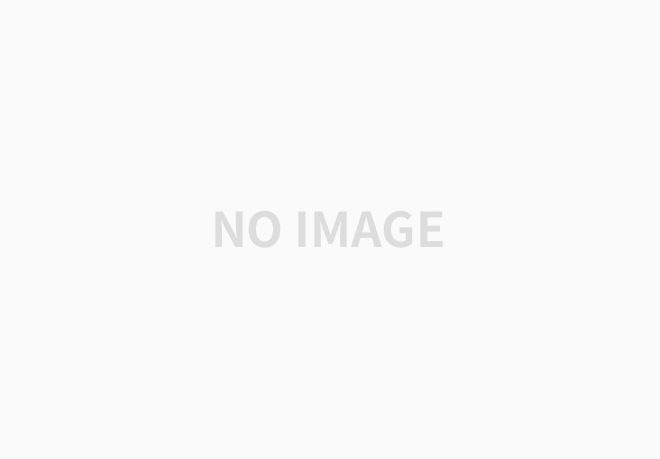
◆ One-Class SVM
- 종속변수 정보가 없는 자료를 요약하는 데, SVM을 사용
◆ SVR
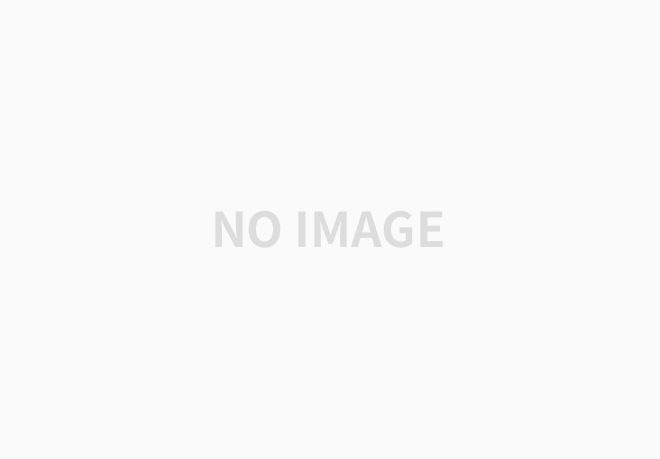
Support vector machine 실습¶
1. 데이터 불러오기, 및 SVM 적합¶
In [1]:
import numpy as np
import matplotlib.pyplot as plt
- 함수 불러오기
In [3]:
from sklearn import svm, datasets
- 모델 적합
In [4]:
iris = datasets.load_iris()
X=iris.data[:,:2]
y=iris.target
C=1
clf=svm.SVC(kernel='linear',C=C)
clf.fit(X,y)
Out[4]:
SVC(C=1, kernel='linear')
In [5]:
from sklearn.metrics import confusion_matrix
y_pred=clf.predict(X)
confusion_matrix(y,y_pred)
Out[5]:
array([[50, 0, 0], [ 0, 38, 12], [ 0, 15, 35]], dtype=int64)
2. kernel SVM 적합 및 비교¶
- LinearSVC
In [6]:
clf=svm.LinearSVC(C=C,max_iter=10000)
clf.fit(X,y)
y_pred=clf.predict(X)
confusion_matrix(y,y_pred)
Out[6]:
array([[49, 1, 0], [ 2, 30, 18], [ 0, 9, 41]], dtype=int64)
- radial basis function
In [7]:
clf=svm.SVC(kernel='rbf',gamma=0.7,C=C,max_iter=10000)
clf.fit(X,y)
y_pred=clf.predict(X)
confusion_matrix(y,y_pred)
Out[7]:
array([[50, 0, 0], [ 0, 37, 13], [ 0, 13, 37]], dtype=int64)
- polynomial kernel
In [9]:
clf=svm.SVC(kernel='poly',degree=3,C=C,gamma='auto')
clf.fit(X,y)
y_pred=clf.predict(X)
confusion_matrix(y,y_pred)
Out[9]:
array([[50, 0, 0], [ 0, 38, 12], [ 0, 16, 34]], dtype=int64)
- 시각적 비교
- 함수 정의
In [10]:
def make_meshgrid(x, y, h=.02):
x_min, x_max = x.min() - 1, x.max() + 1
y_min, y_max = y.min() - 1, y.max() + 1
xx, yy = np.meshgrid(np.arange(x_min, x_max, h),
np.arange(y_min, y_max, h))
return xx, yy
def plot_contours(ax, clf, xx, yy, **params):
Z = clf.predict(np.c_[xx.ravel(), yy.ravel()])
Z = Z.reshape(xx.shape)
out = ax.contourf(xx, yy, Z, **params)
return out
- 데이터 불러오기
In [11]:
iris = datasets.load_iris()
X = iris.data[:, :2]
y = iris.target
- 모델정의 및 피팅
In [12]:
C = 1.0 #Regularization parameter
models = (svm.SVC(kernel='linear', C=C),
svm.LinearSVC(C=C, max_iter=10000),
svm.SVC(kernel='rbf', gamma=0.7, C=C),
svm.SVC(kernel='poly', degree=3, gamma='auto', C=C))
models = (clf.fit(X, y) for clf in models)
In [13]:
titles = ('SVC with linear kernel',
'LinearSVC (linear kernel)',
'SVC with RBF kernel',
'SVC with polynomial (degree 3) kernel')
In [14]:
fig, sub = plt.subplots(2, 2)
plt.subplots_adjust(wspace=0.4, hspace=0.4)
X0, X1 = X[:, 0], X[:, 1]
xx, yy = make_meshgrid(X0, X1)
for clf, title, ax in zip(models, titles, sub.flatten()):
plot_contours(ax, clf, xx, yy,
cmap=plt.cm.coolwarm, alpha=0.8)
ax.scatter(X0, X1, c=y, cmap=plt.cm.coolwarm, s=20, edgecolors='k')
ax.set_xlim(xx.min(), xx.max())
ax.set_ylim(yy.min(), yy.max())
ax.set_xlabel('Sepal length')
ax.set_ylabel('Sepal width')
ax.set_xticks(())
ax.set_yticks(())
ax.set_title(title)
plt.show()
- LinearSVC minimizes the squared hinge loss while SVC minimizes the regular hinge loss.
- LinearSVC uses the One-vs-All (also known as One-vs-Rest) multiclass reduction while SVC uses the One-vs-One multiclass reduction.
In [15]:
from IPython.core.display import display, HTML
display(HTML("<style>.container {width:80% !important;}</style>"))
728x90
'Data scientist > Machine Learning' 카테고리의 다른 글
신경망 모형 + Python_Code (0) | 2021.08.27 |
---|---|
의사결정나무 + Python_Code (0) | 2021.08.26 |
LDA + Python_Code (0) | 2021.08.25 |
K-NN + Python_Code (0) | 2021.08.25 |
Naive Bayes + Python_Code (0) | 2021.08.25 |